11-09-2024
The Role of AI in Clinical Trials: Boosting Efficiency and Reducing Costs
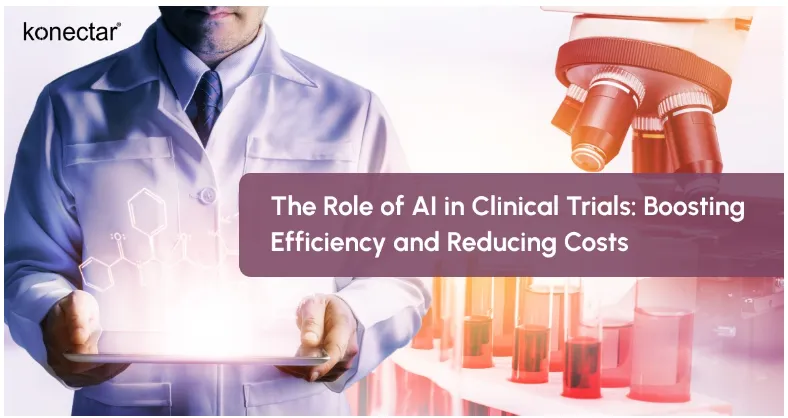
Clinical trials are essential for advancing medical science. They provide the evidence that the newly devised drugs are effective in treating or managing a disease or a condition. However, clinical trials have long been known for being labor-intensive, costly and having multi-year timelines.
However, AI has transformed this aspect of the life sciences industry as well. It has helped streamline clinical trials processes, enhanced precision and improved outcomes. Let us further understand how Ai is revolutionizing patient recruitment, optimizing trial design and protocols, and streamlining data management and analysis.
In this article:
AI in Patient Recruitment and Selection
One of the most challenging aspects of clinical trials is selecting the right participants. Traditional methods can be slow and inefficient. They often rely on manual processes and limited data. AI is addressing these challenges by leveraging advanced technologies to enhance patient recruitment and selection in several key ways. Here’s a look at how AI is driving the change in patient recruiting and selection.
1. Data-Driven Matching
AI algorithms are capable of analyzing vast amounts of patient data from various sources such as electronic health records (EHRs), genetic information, and medical histories. AI systems use natural language processing (NLP) to extract relevant information from unstructured data in EHRs, such as patient notes and lab results. This capability allows researchers to efficiently sift through large datasets and identify potential candidates who might otherwise be overlooked.
This data-driven approach increases the likelihood of finding suitable participants and accelerates the recruitment process.
2. Predictive Analytics
AI-powered predictive analytics play a crucial role in forecasting patient enrollment rates and identifying potential recruitment challenges. By analyzing historical data and trends, AI tools can provide valuable insights into the likely success of recruitment efforts.
Predictive models can estimate how long it will take to recruit a certain number of participants based on factors such as geographic location, patient demographics, and historical enrollment rates. This information allows researchers to optimize their recruitment strategies and allocate resources more effectively.
3. Personalized Outreach
Through machine learning algorithms, AI systems can personalize communication strategies based on individual patient profiles. This personalization can include customized messaging, targeted advertisements, and individualized follow-ups, all of which increase the chances of patient participation.
AI-driven chatbots can engage with potential participants in real-time, answering their questions and providing information about the trial.
Optimizing Trial Design and Protocols
Designing an effective clinical trial involves numerous factors, including defining endpoints, selecting interventions, and establishing protocols. AI is revolutionizing trial design by providing tools and insights that enhance the precision and efficiency of these processes:
1. Algorithmic Trial Design
Clinical trials that use artificial intelligence like machine learning models help researchers to analyze historical outcomes, patient characteristics, and treatment responses to suggest the most effective study designs. This capability allows researchers to create trials that are more likely to yield meaningful results.
2. Simulations and Modeling
AI-powered simulations and modeling can predict how different trial designs might perform. By running virtual trials, researchers can evaluate various scenarios and refine their protocols before implementing them in real-world settings. These simulations can help identify potential issues and optimize trial designs to enhance their effectiveness.
3. Adaptive Trials
AI can also help researchers to facilitate trial designs, where protocols can be adjusted in real-time based on interim results. This flexibility allows researchers to modify the trial in response to emerging data. This leads to improved outcomes and reduction in the time required to reach conclusive results.
4. . Protocol Optimization
By examining data from previous trials and comparing it with current protocols, AI systems can suggest modifications that reduce patient burden, enhance adherence, and streamline data collection processes.
Streamlining Data Management and Analysis
Data management and analysis are central to clinical trials, involving the collection, integration, and interpretation of complex datasets. AI is streamlining these processes, making it easier to handle large volumes of data and extract actionable insights:
1. Automated Data Collection
AI technologies can automate data collection processes, reducing the risk of human error and ensuring consistency. For example, AI-driven tools can extract data from electronic health records (EHRs), wearable devices, and patient-reported outcomes in real-time. This automation not only improves accuracy but also speeds up the data collection process.
Automated data collection also reduces the burden on trial staff, allowing them to focus on more critical tasks. By minimizing manual data entry and validation, AI helps ensure that trial data is accurate and up-to-date.
2. Data Integration
AI can integrate data from various sources, such as clinical notes, laboratory results, and imaging studies, into a unified system. This comprehensive view enables more accurate analysis and facilitates a deeper understanding of patient outcomes.
3. Advanced Analytics
AI algorithms can perform advanced statistical analyses and identify patterns that might be missed using traditional methods. Machine learning models can detect correlations, predict outcomes, and uncover hidden insights from complex datasets. This advanced analytics capability enhances the depth and accuracy of data analysis.
4. Real-Time Monitoring
AI enables real-time monitoring of trial data, allowing researchers to track progress and detect potential issues as they arise. This capability is particularly valuable for ensuring data integrity and making timely decisions based on ongoing results.
Redefining Healthcare: Artificial Intelligence for the Future
The integration of artificial intelligence in pharma industry is transforming the field of medical research. Right from patient recruitment and trial design to data management, AI has helped researchers to enhance the efficiency, accuracy, and overall effectiveness of clinical trials. This has paved the way for more successful and timely advancements in healthcare.
AI is thus not only improving the efficiency of clinical trials but also enabling more precise and personalized approaches to medical research. As AI technology continues to evolve, its role in clinical trials is expected to expand, further revolutionizing the field and accelerating the development of new treatments and therapies. Life sciences teams aiming to gain in-depth insights into the clinical trial landscape and strategically plan their initiatives can leverage konectar Clinical Trial Analytics. For more information, book a meeting with our team today!
FAQs
- How does AI improve patient recruitment for clinical trials?
AI improves patient recruitment by analyzing large datasets to match individuals with trial criteria, predicting enrollment rates and personalizing outreach. This data-driven approach accelerates the recruitment process and enhances the likelihood of finding suitable participants.
- Can AI help in designing clinical trial protocols?
Yes, AI assists in designing clinical trial protocols by proposing optimized designs, running simulations, facilitating adaptive trials, and recommending protocol improvements.
- What are the benefits of using AI for data management in clinical trials?
AI helps in data management by automating data collection, integrating data from various sources, performing advanced analytics, and enabling real-time monitoring. This leads to streamlined data handling, improved accuracy, and provides valuable insights for decision-making.
- Are there any challenges associated with using AI in clinical trials?
Challenges include ensuring data privacy and security, integrating AI tools with existing systems, and the need for continuous validation of AI models.